Latest Posts
In Crypto we Still “Trust”
Most likely, you've heard, seen, or read by now about how cryptocurrency holds the potential to create a society in which the wealthy and...
Blockchain News
In Crypto we Still “Trust”
Most likely, you've heard, seen, or read by now about how cryptocurrency holds the potential to create a society in which the wealthy and...
Understanding why Wall Street likes Crypto
The co-founder of the cryptocurrency education platform "Altcoin Daily," Aaron Arnold, and Roundtable host Rob Nelson recently discussed the notable change in business attitudes about...
AI News
ML News
Discovering chromosome locations in individual cell nucleus through ML
The understanding of how the human genome is arranged within a single cell has advanced significantly thanks to research from Carnegie Mellon University's School...
Data News
The Three Qs for Big Data Insights
Good data can mean the difference between profit and failure in financing. Making wiser choices about who to lend to and how much at what...
Youtube @blockgeni
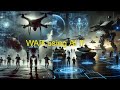
Israel’s use of AI offers a terrifying glimpse at where warfare could be headed
05:55
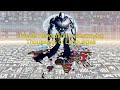
The AI Revolution Is destroying Thousands of Languages
06:40
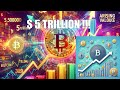
CEO of Ripple predicts crypto market reaching 5 Trillion this year
02:01
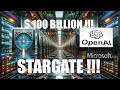
Microsoft and OpenAI are Planning a $100 billion Supercomputer
03:37
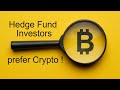
Crypto Options preferred by Goldman’s Hedge Fund Clients
03:20
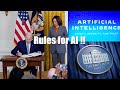
New Guidelines on Government Use of AI
02:43
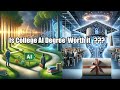
Are College AI Degree Programs Really Worth it ?
03:12
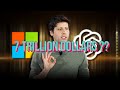
Sam Altman Wants Trillion Dollars to Transform the Chip and AI Business
04:31
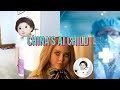
China's 1st AI Child
01:37
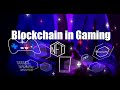
How Blockchain is changing the Gaming industry
04:54
Data DIY
- Advertisement -
AI DIY
Python metaprogramming Tutorial
Metaprogramming, like metadata, is the creation of programs that manipulate other programs. It is widely assumed that metaprograms are programs that generate other programs....
- Advertisement -