Cyber criminals have improved their abilities to compromise systems and get around security measures during the past few decades. As a result, finding and correctly identifying malware is a major problem for many companies and people globally.
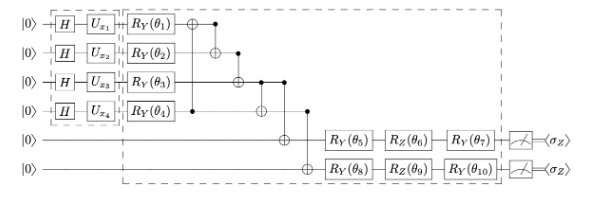
The possibility of machine learning approaches for categorizing malware and determining what steps should be done to eliminate it has lately been investigated by cyber security professionals. Studies revealed that many of these techniques can be deceived or fall short in correctly identifying malware that they have never encountered, despite the fact that some of them have obtained promising outcomes.
Researchers at Orange Innovation Inc. recently conducted a study evaluating the potential of the quantum version of machine learning algorithms in the hopes of discovering more trustworthy methods to categorize malware. Their pre-publication on arXiv study outlines potential research areas for future cyber-security studies and provides some initial insight into the advantages and disadvantages of two categories of quantum machine learning models.
According to co-author of the study Tony Quertier, he has been experimenting with the use of artificial intelligence for malware research since 2019. With Grégoire Barrué, who began his post-doc in October, they wish to investigate the potential contributions that quantum technology might make to this issue. They anticipate being able to use their theoretical skills to better comprehend this topic because they both have backgrounds in mathematics in two complementing areas.
Quantum machine learning, according to Quertier and Barrué, may enable users to get greater knowledge from less amounts of data. They have thus far evaluated the effectiveness of two different quantum machine learning models, known as QSVM and QNN, to test this theory in the context of malware classification.
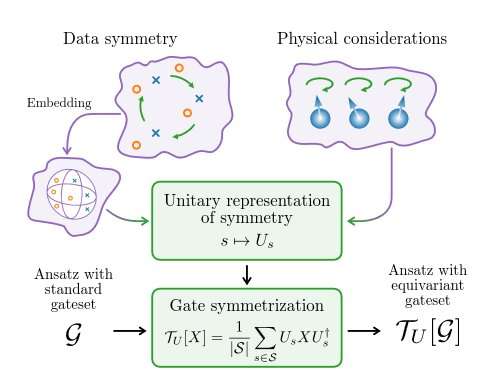
A straightforward QSVM, a quantum adaption of the Support Vector Machines algorithm, is the first method they tested, according to Quertier. Then they experimented with a QNN, a quantum version of a conventional neural network. Considering that they just trained them on a small set of data and used two rather straightforward optimisation techniques at this time (SPSB and data reuploading), they are quite encouraged by the results.
Quertier and BarruĂ© discovered that the QSVM algorithm produced highly encouraging results in their preliminary analyses, exceeding some of the team’s traditional SVMs for malware classification on a number of criteria. The QNN, on the other hand, could classify malware with an accuracy of 87% even though it was simply optimized with data reuploading and a method called SPSB. Given that it was also trained on a small quantity of data, this is extremely impressive.
Naturally, this accuracy is not as good as the conventional algorithms, but Quertier noted that their traditional algorithms are trained on 1 million data whereas here they just used 1,000 samples. This is above and beyond what they anticipated for a first attempt. The capacity of quantum machine learning systems to learn from scant training data is what he finds most intriguing. They now rely far too heavily on having a lot of data and computational power.
Optimizing algorithms to effectively extract more information from a smaller amount of data is the overarching goal of Quertier and BarruĂ©’s ongoing research. Their upcoming research will examine the possibilities of various quantum machine learning techniques, such as quantum convolutional networks (QCCNs), as well as the use of mathematics to optimize and enhance the analysis of the data already available.
For instance, Lie theory can enable them to determine the number of parameters to achieve over parametrization (when the model has enough parameters so that the Fisher Information matrix reaches its maximal rank and therefore has maximal capacity) or even identify symmetries in the data and modify the quantum gates they employ, according to Quertier. A Ph.D. thesis on this topic will begin in October 2023 under the supervision of Daniel Juteau, an expert in this [kind of] mathematics.