A new text-to-speech AI model named VALL-E was unveiled by Microsoft researchers on Thursday. Given a three-second audio sample, VALL-E can accurately mimic a person’s voice. When VALL-E learns a particular voice, it can create audio of that person speaking anything while attempting to capture the speaker’s emotional tone.
According to its developers, VALL-E could be combined with other generative AI models like GPT-3 to create audio content and be used for high-quality text-to-speech applications, speech editing, which would allow a person’s voice to be changed and edited from a text transcript (making them say something they didn’t originally say).
VALL-E, which Microsoft refers to as a “neural codec language model,” is based on a technology known as EnCodec that Meta unveiled in October 2022. VALL-E produces discrete audio codec codes from text and acoustic cues, in contrast to conventional text-to-speech systems that typically synthesis speech by modifying waveforms. It basically listens to a person’s voice, breaks it down into discrete parts (referred to as “tokens”) using EnCodec, and then compares those tokens to training data to determine how that voice would sound if it said additional sentences than the three-second sample. Or, to quote Microsoft from the VALL-E paper:
VALL-E generates the corresponding acoustic tokens conditioned on the acoustic tokens of the 3-second enrolled recording and the phoneme prompt, which confine the speaker and content information, respectively, to synthesize individualized speech (e.g., zero-shot TTS). The final waveform is created using the generated acoustic tokens and the appropriate neural codec decoder.
Microsoft used LibriLight, an audio library put together by Meta, to train VALL- E’s speech synthesis skills. The majority of the 60,000 hours of English-language speech are taken from LibriVox public domain audiobooks and are spoken by more than 7,000 different people. The voice in the three-second sample must closely resemble a voice in the training data for VALL-E to get a satisfactory result.
Microsoft makes a tonne of audio examples of the VALL-E AI model in action available on the webpage for the example. The “Speaker Prompt,” one of the samples, is a three-second auditory cue that VALL-E must duplicate. For comparative purposes, the “Ground Truth” is a previously recorded excerpt of the same speaker using a certain phrase (sort of like the “control” in the experiment). The “Baseline” sample represents a typical text-to-speech synthesis example, and the “VALL-E” sample represents the output of the VALL-E model.
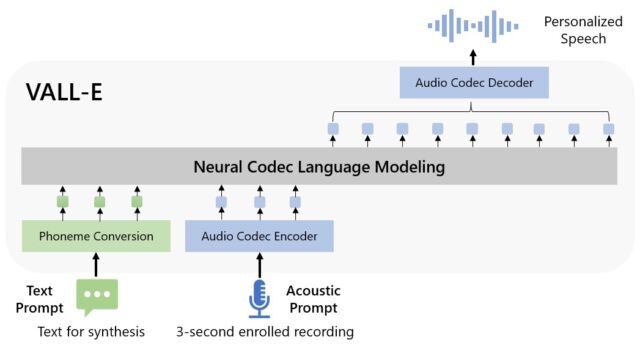
The researchers provided VALL-E merely the three-second “Speaker Prompt” sample plus a text string (what they wanted the voice to say) in order to produce those results. Therefore, contrast the samples from “Ground Truth” and “VALL-E.” The two examples are extremely similar in certain instances. It is the intention of the model that some VALL-E findings be mistaken for human speech rather than appearing to be computer-generated.
The “acoustic environment” of the sample audio can be replicated by VALL-E in addition to maintaining the vocal timbre and emotional tone of the speaker. The audio output, for instance, will imitate the acoustic and frequency qualities of a telephone call in its synthesized output, which is a fancy way of stating that it will sound like a telephone call as well. Additionally, Microsoft’s samples (included in the “Synthesis of Diversity” section) show how VALL-E can produce different voice tones by altering the random seed utilized during generation.
We were unable to evaluate VALL-E’s capabilities since Microsoft has not made the VALL-E code available for others to experiment with, maybe because of VALL-E’s potential to cause trouble and deception. The potential social harm that this technology can do seems to be recognized by the researchers. They state in the paper’s conclusion:
“VALL-E may carry potential hazards if the model is misused, such as spoofing voice identification or impersonating a specific speaker, as it may synthesise speech that maintains speaker identity. Building a detection algorithm to determine whether an audio clip was created using VALL-E will help to reduce these hazards. When advancing the models, we’ll also take Microsoft AI Principles into consideration.”