
Permafrost (permafrost for more than 2 years) occupies most of the earth and cover about 15% of the Northern Hemisphere.
Permafrost is important to our climate as it contains large amounts of biomass stored as methane and carbon dioxide and uses the tundra floor as a carbon sink.
It is important to our climate as it contains large amounts of biomass stored as methane and carbon dioxide and uses the tundra floor as a carbon sink. However, permafrost’s innate traits and converting nature aren’t extensively understood.
As global warming warms the earth and melts the soil, it is expected that the carbon cycle of permafrost will accelerate, releasing greenhouse gases contained in the soil into the atmosphere and creating a feedback loop that exacerbates climate change.
Remote sensing is one way to understand the width, dynamics, and changes of the permafrost layer. Candi Witarana, an assistant professor of natural resources and the environment at the University of Connecticut, said: “Satellite imagery helps us monitor remote landscapes in unprecedented ways.”
Over the last two decades, much of the Arctic Circle has been very accurately mapped by commercial satellites. These maps are a treasure trove of data about this largely unexplored area. But the data is so big and awkward that science is difficult, says Withalana.
With funding and support from the National Science Foundation (NSF) under the navigation of the new Arctic program, Withalana will join Kenton McHenry of the National Institute of Supercomputer Applications and the Arctic of the Woodwell Climate Research Center. Along with the sphere researcher Anna Lilledal, the Arctic Permanent Frozen Soil is much more accessible.
The team had free access to an archive of over 1 million image scenes captured in the Arctic Circle. Traditional analysis and feature extraction methods have failed because this is a large amount of data. “Here, we used AI-based deep learning techniques to process and analyze this large amount of data,” says Witharana.
One of the most distinctive and easy-to-understand features of permafrost is the ice wedge, which creates polygons that can be recognized in satellite images.
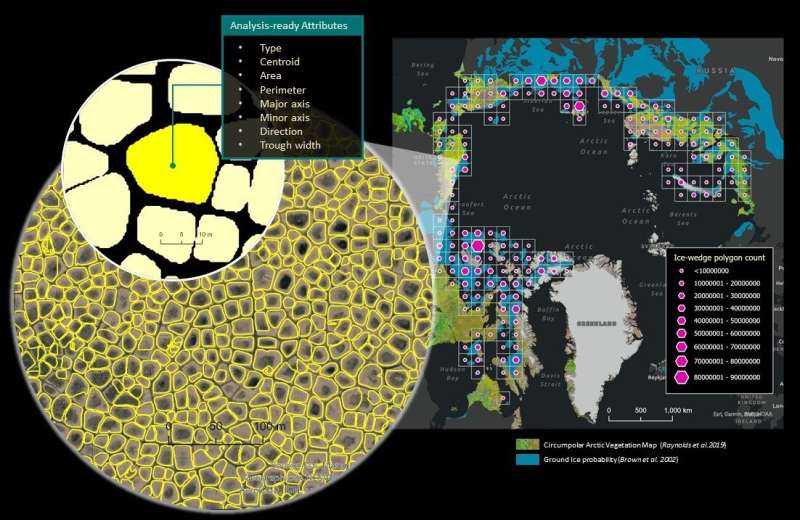
“The ice wedge is formed from the freezing and thawing of the tundra soil,” said Liljedahl. “Some of them are tens of thousands of years old.”
The shape and dimensions of an ice vein polygon can provide important information about the condition and rate of change of an area. But they circumvent traditional analysis.
I was on Facebook a few years ago and found out that they started using software to recognize faces in photos,” Liljedal recalls. “I wonder if this could apply to venous landfills in the Arctic.”
She contacted Withalana and McHenry, whom she met at a panel meeting in Washington, D.C. She met her and invited her to participate in her project ideas. They each provided complementary skills in domain expertise, code development, and big data management.
Starting in 2018, Witharana started using neural networks to detect not friends’ faces but polygons from thousands of satellite images of the North Pole. To do this, Witharana and his team first had to annotate 50,000 individual polygons, hand-draw their contours, and classify them as low center or high center.
Deep-centered ice wedge polygons form a pool in the center of the ribbed outer part. According to Rishdar, the high-centered ice wedge looks like a muffin, proof that the ice wedge melts. The two types have distinct structural hydrological characteristics that are important to understand in terms of their role in climate change and to plan the future infrastructure of the Arctic community.
“Permafrost is not characterized by these spatial-scale climate models,” says Richedal. “This study helps us to derive a baseline and see how changes occur over time.”
They trained the model with annotated images, fed satellite images into a neural network, and tested them with unannotated data. There was the first challenge. For example, images trained for Canada were less effective in Russia, where the ice wedges are old and different in shape. However, after three years, the team’s accuracy rate will be 80-90%.
They have described the results of this research in the ISPRS Journal of Photogrammetry and Remote Sensing (2020), the Journal of Imaging (2020) and Remote Sensing (2021).
After demonstrating that deep learning techniques work, they focus on the Longhorn supercomputer (a GPU-based IBM system that can quickly perform AI inference tasks) and Pittsburgh’s Bridges 2 system operated by the Texas Advanced Computing Center (TACC). did. A supercomputing center assigned by the NSFfunded Extreme Science and Engineering Discovery Environment (XSEDE) to analyze data.
By the end of 2021, the team had identified and mapped 1.2 billion ice wedge-shaped polygons in satellite data. They estimated that they were about half way through the entire dataset.
Each individual image analysis includes pre-processing (to improve image clarity and remove non-ground features such as lakes), processing (where polygons are detected and featured), and post-processing
In addition to identifying and classifying ice wedge polygons, this method also obtains information about the wedge size, dip size, and other characteristics.
Individual analysis can be performed within an hour. However, the number of them is so large that it is impossible to run them anywhere other than a large supercomputer that can calculate in parallel.
Vitarana and her team recently tested the workflow to find the optimal configuration for efficient supercomputing. In 2022, the journal Photogrammetric Engineering and Remote Sensing (PE&RS) evaluated four workflow schemes on two different HPC systems and found optimal settings for high-speed analysis. A separate 2022 have a look at in PE&RS explored the efficacy of various picture augmentation methods (including converting the hue or saturation) while implemented to deep studying convolutional neural internet algorithms to understand ice-wedge polygons from industrial satellite tv for pc imagery.
A separate 2022 have a look at in PE&RS explored the efficacy of various picture augmentation methods (including converting the hue or saturation) while implemented to deep studying convolutional neural internet algorithms to understand ice-wedge polygons from industrial satellite tv for pc imagery. Too many quick changes. We need to be able to understand and report on what is going on in the permafrost.”
Instead of waiting ten years to learn something, they can learn about it right away and explore it on their own. Another important phase of the research project takes place when researchers analyze satellite images of different years and seasons. Comparing the states of the ice wedge polygons can reveal trends and trajectories. The speed of landscape change and where these changes intersect villages and infrastructure.
This is a perfect example of how previous investments in computing infrastructure and a new understanding of deep learning technology are building resources to help with critical Arctic problems. ” Ken Drama Clauclan says. With the help of earth observation technology, you can see how climate change is happening, and even the land is changing. It is the primary tool for monitoring, monitoring, forecasting and making decisions to prevent adverse effects on vulnerable areas. ”