China’s RUDN University chemists and associates developed a number of machine learning models and identified a class of possible medications that inhibit the enzyme in charge of uncontrolled cell development. The findings were released in Biomedicines.
An enzyme called cyclin-dependent kinase 2 (CDK 2) is involved in the control of cell division. Although CDK 2 is not required for the development of healthy cells, it is extremely important for the unchecked expansion of cancer cells. Finding potent CDK 2 inhibitors is crucial because it can stop tumor growth by inhibiting CDK 2 activity. For this, chemists from RUDN University and their Chinese counterparts merged machine learning and molecular modelling. As a result, numerous possible inhibitors were found by chemists.
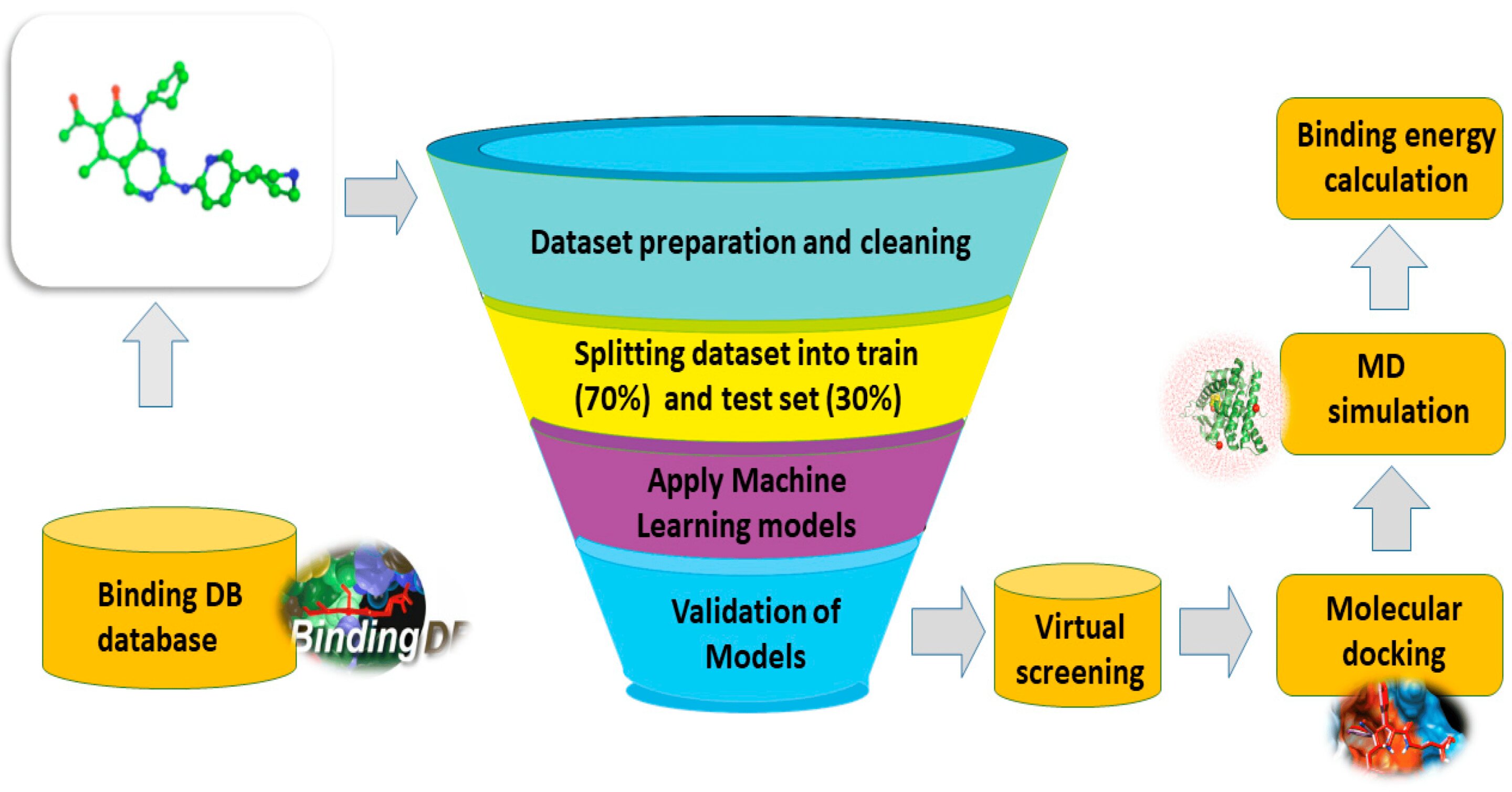
A prospective target for the therapy of cancer is cyclin-dependent kinase 2. It is crucial for anticancer therapy that its inhibitors are developed. Although further research is needed to determine how this enzyme contributes to tumor development, it is already known that its inhibition is effective in the treatment of cancer. According to Alexander Novikov, Ph.D. in Chemistry, senior researcher at the Joint Institute of Chemical Research of RUDN University, several inhibitors have already undergone clinical trials, but a selective inhibitor especially for this enzyme has not yet been discovered.
Chemists employed machine learning techniques to discover a candidate drug. To identify effective CDK 2 inhibitors, the authors created a number of models. The researchers created a molecular model using the molecular docking technique, which can pinpoint the ideal orientation for molecules to form stable complex.
Using machine learning methods, 25 possible active CDK 2 inhibitors were found with 98% accuracy. By employing molecular docking, chemists evaluated each one. The most effective drugs were three. The molecular dynamics method was used to create a computer simulation for the top three, which was then contrasted with the reference substance dalpiciclib. It was discovered that all three were more compact and stable.
The three computed compounds had more stable behavior and compactness as compared to the control medication dalpiciclib. Despite the encouraging findings, our study includes a number of drawbacks. To verify inhibitory action and possible therapeutic efficacy, comprehensive in vitro and in vivo clinical investigations are required. The impact of substances on off-target interactions and their toxicity will also need to be studied when designing medications, according to Alexander Novikov, Ph.D. in Chemistry, senior researcher at the Joint Institute of Chemical Research of RUDN University.