AI systems with emotional intelligence could learn faster and be more helpful
In the past year, have you found yourself under stress? Have you ever wished for help coping? Imagine if, throughout the pandemic, you’d had a virtual therapist powered by an artificial intelligence (AI) system, an entity that empathized with you and gradually got to know your moods and behaviors. Therapy is just one area where we think an AI system that can recognize and interpret emotions could offer great benefits to people.
Our team hails from Microsoft’s Human Understanding and Empathy group, where our mission is to imbue technology with emotional intelligence. Why? With that quality, AI can better understand its users, more effectively communicate with them, and improve their interactions with technology. The effort to produce emotionally intelligent AI builds on work in psychology, neuroscience, human-computer interaction, linguistics, electrical engineering, and machine learning.
Lately, we’ve been considering how we could improve AI voice assistants such as Alexa and Siri, which many people now use as everyday aides. We anticipate that they’ll soon be deployed in cars, hospitals, stores, schools, and more, where they’ll enable more personalized and meaningful interactions with technology. But to achieve their potential, such voice assistants will require a major boost from the field of affective computing. That term, coined by MIT professor Rosalind W. Picard in a 1997 book by the same name, refers to technology that can sense, understand, and even simulate human emotions. Voice assistants that feature emotional intelligence should be more natural and efficient than those that do not.
Consider how such an AI agent could help a person who’s feeling overwhelmed by stress. Currently, the best option might be to see a real human psychologist who, over a series of costly consultations, would discuss the situation and teach relevant stress-management skills. During the sessions, the therapist would continually evaluate the person’s responses and use that information to shape what’s discussed, adapting both content and presentation in an effort to ensure the best outcome.
While this treatment is arguably the best existing therapy, and while technology is still far from being able to replicate that experience, it’s not ideal for some. For example, certain people feel uncomfortable discussing their feelings with therapists, and some find the process stigmatizing or time-consuming. An AI therapist could provide them with an alternative avenue for support, while also conducting more frequent and personalized assessments. One recent review article found that 1 billion people globally are affected by mental and addictive disorders; a scalable solution such as a virtual counselor could be a huge boon.
There’s some evidence that people can feel more engaged and are more willing to disclose sensitive information when they’re talking to a machine. Other research, however, has found that people seeking emotional support from an online platform prefer responses coming from humans to those from a machine, even when the content is the same. Clearly, we need more research in this area.
In any case, an AI therapist offers a key advantage: It would always be available. So it could provide crucial support at unexpected moments of crisis or take advantage of those times when a person is in the mood for more analytical talk. It could potentially gather much more information about the person’s behavior than a human therapist could through sporadic sessions, and it could provide reminders to keep the person on track. And as the pandemic has greatly increased the adoption of telehealth methods, people may soon find it quite normal to get guidance from an agent on a computer or phone display.
For this kind of virtual therapist to be effective, though, it would require significant emotional intelligence. It would need to sense and understand the user’s preferences and fluctuating emotional states so it could optimize its communication. Ideally, it would also simulate certain emotional responses to promote empathy and better motivate the person.
The virtual therapist is not a new invention. The very first example came about in the 1960s, when Joseph Weizenbaum of MIT wrote scripts for his ELIZA natural-language-processing program, which often repeated users’ words back to them in a vastly simplified simulation of psychotherapy. A more serious effort in the 2000s at the University of Southern California’s Institute for Creative Technologies produced SimSensei, a virtual human initially designed to counsel military personnel. Today, the most well-known example may be Woebot, a free chatbot that offers conversations based on cognitive behavioral therapy. But there’s still a long way to go before we’ll see AI systems that truly understand the complexities of human emotion.
Our group is doing foundational work that will lead to such sophisticated machines. We’re also exploring what might happen if we build AI systems that are motivated by something approximating human emotions. We argue that such a shift would take modern AI’s already impressive capabilities to the next level.
Only a decade ago, affective computing required custom-made hardware and software, which in turn demanded someone with an advanced technical degree to operate. Those early systems usually involved awkwardly large sensors and cumbersome wires, which could easily affect the emotional experience of wearers.
Today, high-quality sensors are tiny and wireless, enabling unobtrusive estimates of a person’s emotional state. We can also use mobile phones and wearable devices to study visceral human experiences in real-life settings, where emotions really matter. And instead of short laboratory experiments with small groups of people, we can now study emotions over time and capture data from large populations “in the wild,” as it were.
To predict someone’s emotional state, it’s best to combine readouts. In this example, software that analyzes facial expressions detects visual cues, tracking the subtle muscle movements that can indicate emotion (1). A physiological monitor detects heart rate (2), and speech-recognition software transcribes a person’s words and extracts features from the audio (3), such as the emotional tone of the speech.Earlier studies in affective computing usually measured emotional responses with a single parameter, like heart rate or tone of voice, and were conducted in contrived laboratory settings. Thanks to significant advances in AI—including automated speech recognition, scene and object recognition, and face and body tracking—researchers can do much better today. Using a combination of verbal, visual, and physiological cues, we can better capture subtleties that are indicative of certain emotional states.
We’re also building on new psychological models that better explain how and why people express their emotions. For example, psychologists have critiqued the common notion that certain facial expressions always signal certain emotions, arguing that the meaning of expressions like smiles and frowns varies greatly according to context, and also reflects individual and cultural differences. As these models continue to evolve, affective computing must evolve too.
This technology raises a number of societal issues. First, we must think about the privacy implications of gathering and analyzing people’s visual, verbal, and physiological signals. One strategy for mitigating privacy concerns is to reduce the amount of data that needs to leave the sensing device, making it more difficult to identify a person by such data. We must also ensure that users always know whether they’re talking to an AI or a human. Additionally, users should clearly understand how their data is being used—and know how to opt out or to remain unobserved in a public space that might contain emotion-sensing agents.
As such agents become more realistic, we’ll also have to grapple with the “uncanny valley” phenomenon, in which people find that somewhat lifelike AI entities are creepier than more obviously synthetic creatures. But before we get to all those deployment challenges, we have to make the technology work.
As a first step toward an AI system that can support people’s mental health and well-being, we created Emma, an emotionally aware phone app. In one 2019 experiment, Emma asked users how they were feeling at random times throughout the day. Half of them then got an empathetic response from Emma that was tailored to their emotional state, while the other half received a neutral response. The result: Those participants who interacted with the empathetic bot more frequently reported a positive mood.
In a second experiment with the same cohort, we tested whether we could infer people’s moods from basic mobile-phone data and whether suggesting appropriate wellness activities would boost the spirits of those feeling glum. Using just location (which gave us the user’s distance from home or work), time of day, and day of the week, we were able to predict reliably where the user’s mood fell within a simple quadrant model of emotions.
Depending on whether the user was happy, calm, agitated, or sad, Emma responded in an appropriate tone and recommended simple activities such as taking a deep breath or talking with a friend. We found that users who received Emma’s empathetic urgings were more likely to take the recommended actions and reported greater happiness than users who received the same advice from a neutral bot.
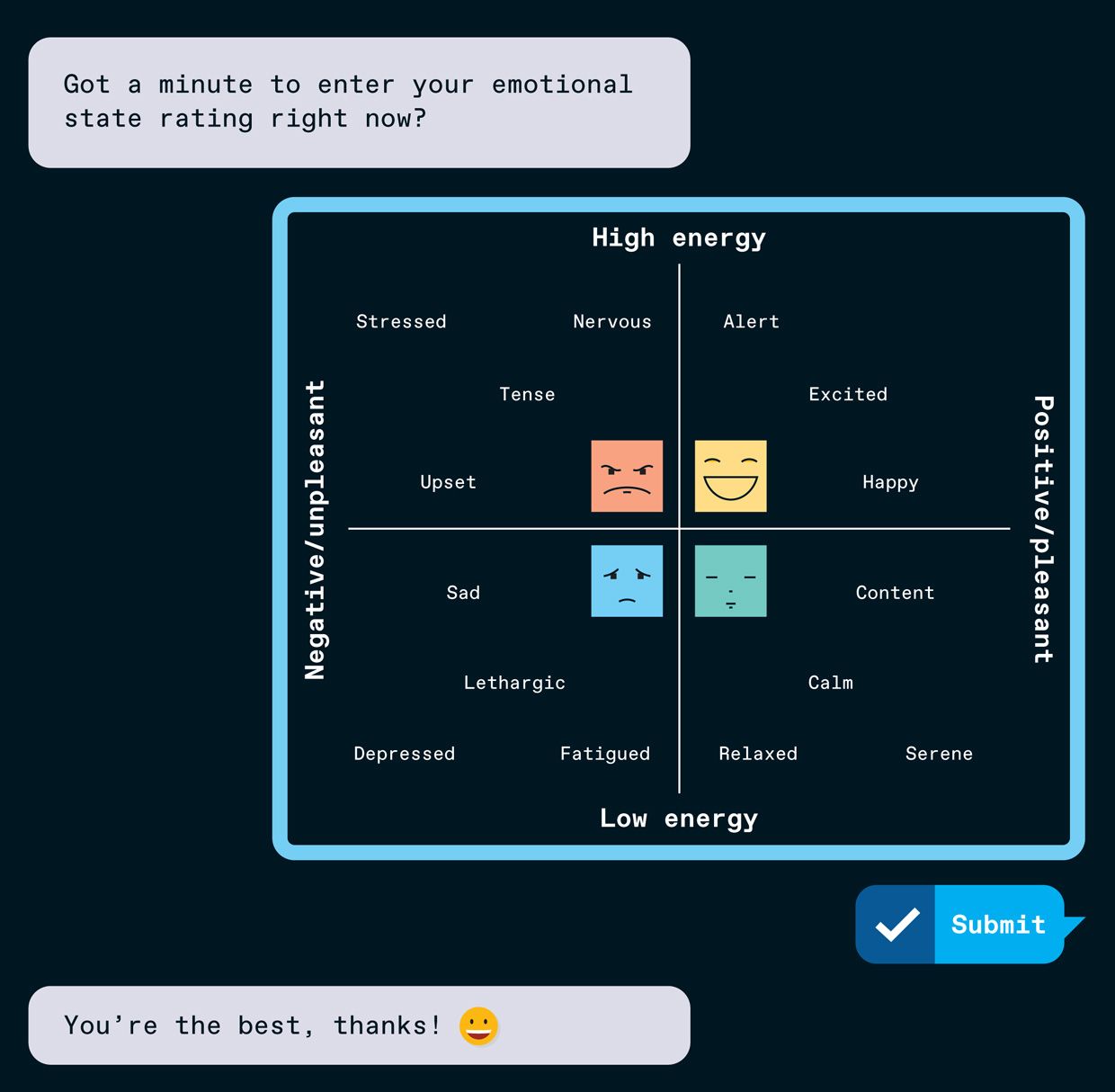
We collected other data, too, from the mobile phone: Its built-in accelerometer gave us information about the user’s movements, while metadata from phone calls, text messages, and calendar events told us about the frequency and duration of social contact. Some technical difficulties prevented us from using that data to predict emotion, but we expect that including such information will only make assessments more accurate.
In another area of research, we’re trying to help information workers reduce stress and increase productivity. We’ve developed many iterations of productivity support tools, the most recent being our work on “focus agents.” These assistants schedule time on users’ calendars to focus on important tasks. Then they monitor the users’ adherence to their plans, intervene when distractions pop up, remind them to take breaks when appropriate, and help them reflect on their daily moods and goals. The agents access the users’ calendars and observe their computer activity to see if they’re using applications such as Word that aid their productivity or wandering off to check social media.
To see whether emotional intelligence would improve the user experience, we created one focus agent that appeared on the screen as a friendly avatar. This agent used facial-expression analysis to estimate users’ emotions, and relied on an AI-powered dialogue model to respond in appropriate tones.
We compared this avatar agent’s impact with that of an emotionless text-based agent and also with that of an existing Microsoft tool that simply allowed users to schedule time for focused work. We found that both kinds of agents helped information workers stay focused and that people used applications associated with productivity for a larger percentage of their time than did their colleagues using the standard scheduling tool. And overall, users reported feeling the most productive and satisfied with the avatar-based agent.
Our agent was adept at predicting a subset of emotions, but there’s still work to be done on recognizing more nuanced states such as focus, boredom, stress, and task fatigue. We’re also refining the timing of the interactions so that they’re seen as helpful and not irritating.
We found it interesting that responses to our empathetic, embodied avatar were polarized. Some users felt comforted by the interactions, while others found the avatar to be a distraction from their work. People expressed a wide range of preferences for how such agents should behave. While we could theoretically design many different types of agents to satisfy many different users, that approach would be an inefficient way to scale up. It would be better to create a single agent that can adapt to a user’s communication preferences, just as humans do in their interactions.
For example, many people instinctively match the conversational style of the person they’re speaking with; such “linguistic mimicry” has been shown to increase empathy, rapport, and prosocial behaviors. We developed the first example of an AI agent that performs this same trick, matching its conversational partner’s habits of speech, including pitch, loudness, speech rate, word choice, and statement length. We can imagine integrating such stylistic matching into a focus agent to create a more natural dialogue.
We’re always talking with Microsoft’s product teams about our research. We don’t yet know which of our efforts will show up in office workers’ software within the next five years, but we’re confident that future Microsoft products will incorporate emotionally intelligent AI.
AI systems that can predict and respond to human emotions are one thing, but what if an AI system could actually experience something akin to human emotions? If an agent was motivated by fear, curiosity, or delight, how would that change the technology and its capabilities? To explore this idea, we trained agents that had the basic emotional drives of fear and happy curiosity.
With this work, we’re trying to address a few problems in a field of AI called reinforcement learning, in which an AI agent learns how to do a task by relentless trial and error. Over millions of attempts, the agent figures out the best actions and strategies to use, and if it successfully completes its mission, it earns a reward. Reinforcement learning has been used to train AI agents to beat humans at the board game Go, the video game StarCraft II, and a type of poker known as Texas Hold’em.
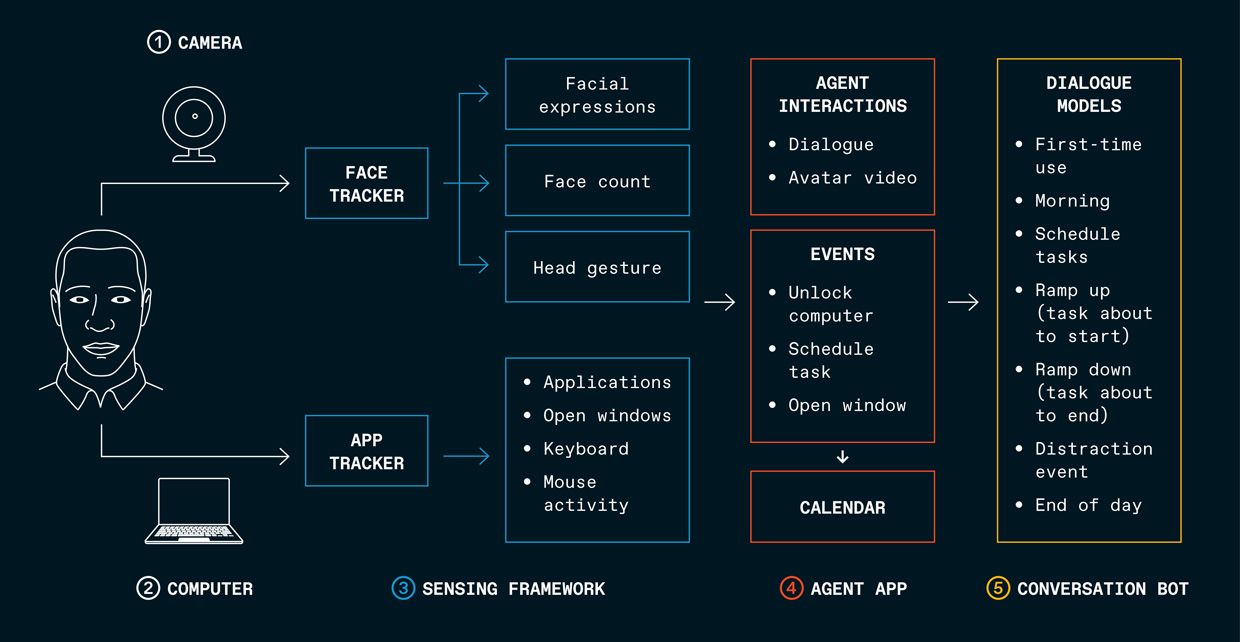
While this type of machine learning works well with games, where winning offers a clear reward, it’s harder to apply in the real world. Consider the challenge of training a self-driving car, for example. If the reward is getting safely to the destination, the AI will spend a lot of time crashing into things as it tries different strategies, and will only rarely succeed. That’s the problem of sparse external rewards. It might also take a while for the AI to figure out which specific actions are most important—is it stopping for a red light or speeding up on an empty street? Because the reward comes only at the end of a long sequence of actions, researchers call this the credit-assignment problem.
Now think about how a human behaves while driving. Reaching the destination safely is still the goal, but the person gets a lot of feedback along the way. In a stressful situation, such as speeding down the highway during a rainstorm, the person might feel his heart thumping faster in his chest as adrenaline and cortisol course through his body. These changes are part of the person’s fight-or-flight response, which influences decision making. The driver doesn’t have to actually crash into something to feel the difference between a safe maneuver and a risky move. And when he exits the highway and his pulse slows, there’s a clear correlation between the event and the response.
We wanted to capture those correlations and create an AI agent that in some sense experiences fear. So we asked people to steer a car through a maze in a simulated environment, measured their physiological responses in both calm and stressful moments, then used that data to train an AI driving agent. We programmed the agent to receive an extrinsic reward for exploring a good percentage of the maze, and also an intrinsic reward for minimizing the emotional state associated with dangerous situations.
We found that combining these two rewards created agents that learned much faster than one that received only the typical extrinsic reward. These agents also crashed less often. What we found particularly interesting, though, is that an agent motivated primarily by the intrinsic reward didn’t perform very well: If we dialed down the external reward, the agent became so risk averse that it didn’t try very hard to accomplish its objective.
During another effort to build intrinsic motivation into an AI agent, we thought about human curiosity and how people are driven to explore because they think they may discover things that make them feel good. In related AI research, other groups have captured something akin to basic curiosity, rewarding agents for seeking novelty as they explore a simulated environment. But we wanted to create a choosier agent that sought out not just novelty but novelty that was likely to make it “happy.”
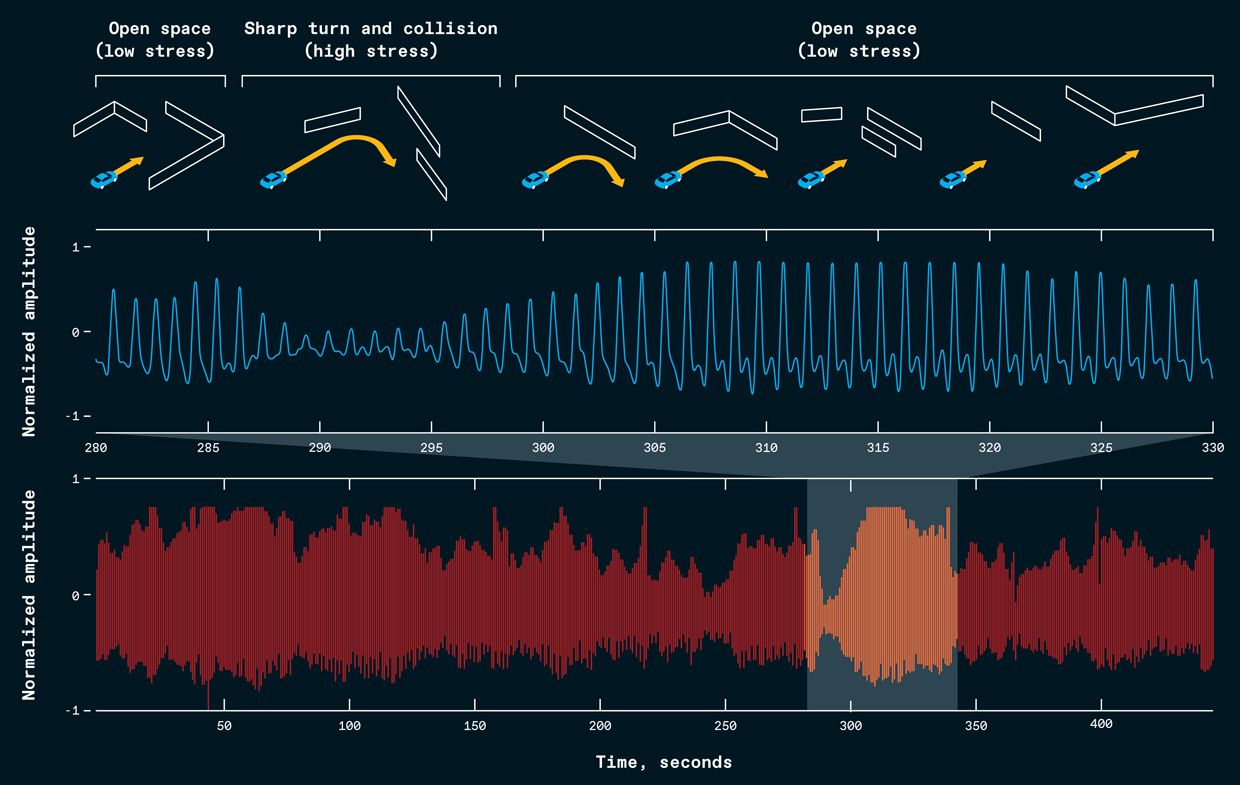
To gather training data for such an agent, we asked people to drive a virtual car within a simulated maze of streets, telling them to explore but giving them no other objectives. As they drove, we used facial-expression analysis to track smiles that flitted across their faces as they navigated successfully through tricky parts or unexpectedly found the exit of the maze. We used that data as the basis for the intrinsic reward function, meaning that the agent was taught to maximize situations that would make a human smile. The agent received the external reward by covering as much territory as possible.
Again, we found that agents that incorporated intrinsic drive did better than typically trained agents—they drove in the maze for a longer period before crashing into a wall, and they explored more territory. We also found that such agents performed better on related visual-processing tasks, such as estimating depth in a 3D image and segmenting a scene into component parts.
We’re at the very beginning of mimicking human emotions in silico, and there will doubtless be philosophical debate over what it means for a machine to be able to imitate the emotional states associated with happiness or fear. But we think such approaches may not only make for more efficient learning, they may also give AI systems the crucial ability to generalize.
Today’s AI systems are typically trained to carry out a single task, one that they might get very good at, yet they can’t transfer their painstakingly acquired skills to any other domain. But human beings use their emotions to help navigate new situations every day; that’s what people mean when they talk about using their gut instincts.
We want to give AI systems similar abilities. If AI systems are driven by humanlike emotion, might they more closely approximate humanlike intelligence? Perhaps simulated emotions could spur AI systems to achieve much more than they would otherwise. We’re certainly curious to explore this question—in part because we know our discoveries will make us smile.
This article has been published from the source link without modification to the text. Only the headline has been changed.