Cody Lloyd’s interest with the Manhattan Project—the US effort to enhance nuclear technology in order to win World War II—led him to pursue a career in nuclear engineering. Lloyd currently trains computers to analyze data from footage of nuclear weapons tests from the 1950s and early 1960s as a research associate in nuclear forensics at the Department of Energy’s Oak Ridge National Laboratory, incorporating his childhood curiosity into his work.
The United States sought to learn more about what happened after a nuclear weapon was used after World War II. Researchers ran tests in the Pacific Ocean and the southwest United States, and they captured the tests on film. The first reel-to-reel videos were used by scientists to manually measure data from the explosions. The films were stored at Los Alamos National Laboratory over the years until they were recently converted into high-resolution digital photographs as part of a project led by Greg Spriggs at Lawrence Livermore National Laboratory in partnership with LANL.
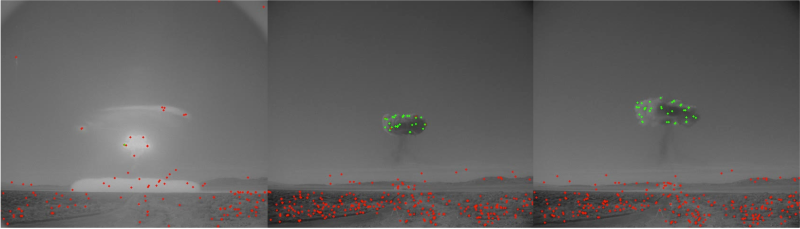
In his work as a nuclear forensic scientist, Lloyd combines cutting-edge computational methods with historical records of nuclear testing to provide invaluable insights into the physics of these kinds of events, which are otherwise difficult to investigate experimentally. He is autonomously extracting information from the blast picture using machine learning methods. After some practice, the algorithms can take a few video frames as input and produce the data he need.
According to Lloyd, the computer can track movement from frame to frame in the movie to demonstrate how the cloud expands and moves through the atmosphere shortly after detonation. This knowledge can be used by scientists to improve models for air movement and dispersion, cloud increase, and how a contaminant might behave if it were released close to a community.
Machine learning models are trained by researchers at DOE labs using measurements manually gathered from the films. Researchers get deeper understanding of how computers perceive the fluid dynamics of blast plumes by comparing measurements with old data. These algorithms can scan a collection of photos and quickly discover the relevant information, saving researchers the time and effort of going through each frame of a film individually. As a cloud rises, for instance, the algorithms can swiftly gauge height and distance as the shape changes over time.
Training data is needed to teach machine learning algorithms since it demonstrates to the computer what kinds of data are necessary to identify in order to respond to a given question. Before presenting the computer with an independent data set to assess the precision of its predictions, researchers often subject the computer to a trained set of images. The historical atmospheric test videos serve as both a training and testing tool because they are the only source of such information. Lloyd chose frames that, although being different from the images the computer needed to answer the study question, yet gave the computer sufficient information.
The films’ quality is one of the project’s difficulties. Videos from seven decades ago might not have the best qualities even though the files are in high resolution. Images from the period of atmospheric testing are on physical film, which means they are vulnerable to additional physical flaws and effects in contrast to the digital images we see today. The backgrounds and explosions in the majority of the movie are starkly contrasted in black and white. Filters are frequently applied to cameras by photographers to make the background appear much darker than it actually is. The solarization effect can cause a fireball to sometimes seem as a brilliant ring with a dark center, while in reality the fireball is always bright. Due to too much brilliant light leaking through the camera’s cracks, some frames are overexposed.
To improve the algorithms’ accuracy, Lloyd incorporated additional historical information, such as the cameras’ location in respect to the explosion. To find out more about the atmospheric factors influencing how the cloud developed and drifted, he downloaded meteorological data. The algorithms can produce better outcomes by receiving more data.
How well pre-built MATLAB and Python machine learning models have worked is one thing that has surprised Lloyd. He is interested in creating his own algorithms to improve feature extraction as he considers potential future uses for this footage analysis.
Lloyd is optimistic about what the future holds. Given that open-air nuclear weapon testing took been in the past and is not expected to happen again, he claimed there are countless applications for the videos. Even though this data is outdated, it is still extremely helpful to comprehend the fallout of material that is released into the air, including where it goes and what it looks like when it hits the ground.
The NNSA Office of Nuclear Detonation Detection – Forensics is funding this research.